Equity in behavioral healthcare: How AI is helping
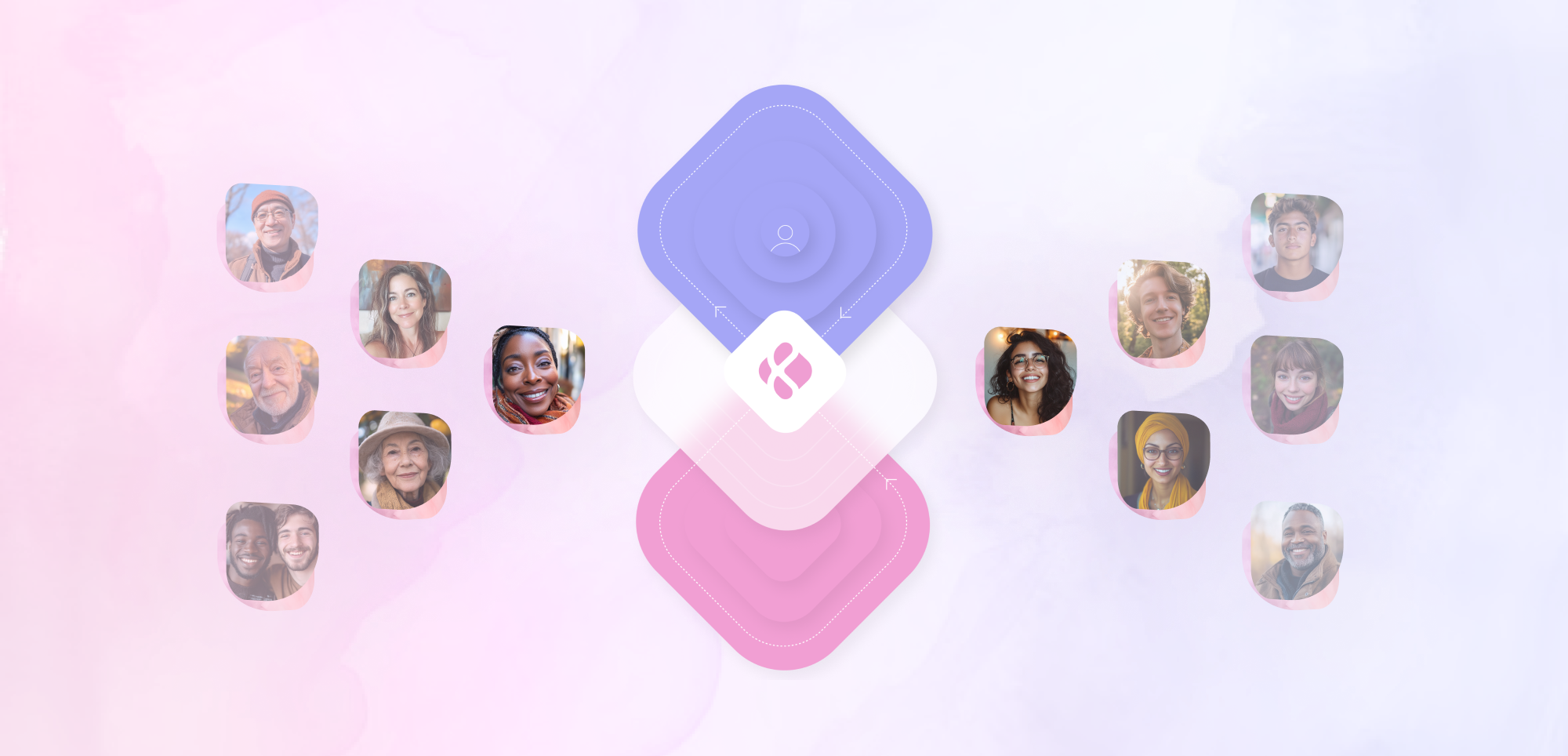
As is the case with healthcare in general, access for mental health support varies across different racial and demographic groups.
This isn’t opinion; it’s math. A 2023 report from the Kaiser Family Foundation found that, while 52% of white adults with diagnosable mental health issues received professional support, that figure stood at just 39% for Black adults, 36% for Hispanic adults, and 25% for Asian adults.
Issues like access, affordability, systemic bias, and cultural attitudes about mental health drive huge disparities in who seeks behavioral health support, who has access, and what type of care they receive.
Recent research in Nature Medicine has shown that clinical AI tools can be hugely beneficial in overcoming some of these barriers—to the tune of a 40% increase in Black and Asian individuals accessing care and a massive 179% increase among non-binary individuals.
That 2024 study—analyzing 129,400 patients across multiple sites—looked at mental health clinics that used Limbic’sclinical AI agent for intake, triage, and assessments. And while the researchers found increases in care access across multiple demographic groups, the differences were most pronounced among historically underrepresented communities.
So what’s happening here? What can these findings tell us about unequal access to mental health care and about how AI might help us address them? Let’s start by looking at the broader issues of access and outcomes.
Stigma as a Driver of Mental Health Disparities in Minority Populations
Numerous studies have documented systemic issues like affordability, geography, and lack of diversity amongst clinical workers. Minority populations disproportionately lack the means to pay for or even have physical proximity to mental health services.
Those who can overcome these hurdles often struggle to find mental health providers representative of their race, ethnicity, or sexual identities, which can discourage people from seeking care.
Hanging over all of this is stigma, both real and perceived. While it is difficult to objectively quantify stigma, researchers have repeatedly found that cultural attitudes toward mental illness are a significant barrier to care. And a 2020 meta-analysis of 29 separate studies covering nearly 200,000 subjects concluded that “Racial minorities showed more stigma than racial majorities for common mental disorders.”
In Black communities, there is sometimes a perception that seeking help reflects personal weakness or even a lack of religious faith. And in some Asian cultures, mental illness can be perceived as a source of shame, not only for the individual but for the family.
For LGBTQ+ individuals, stigma and discrimination can exacerbate mental health challenges—in a population that experiences such challenges at higher rates than other groups. For example, transgender and nonbinary youth are two to 2.5 times more likely to experience depression or suicidality than their cis-gendered peers. And many report feeling unwelcome or misunderstood by healthcare providers—a perception backed by academic research—further deterring them from seeking help.
How AI is Addressing Mental Health Equity
Clinical AI tools offer promising solutions to these inequities by addressing barriers in access, personalization, and representation.
Navigating bias
To err isn’t only human. Everyone should be aware of—and fighting to correct—bias in AI systems. (Limbic is leading the way here.) AI has the potential to miss social cues and misinterpret cultural influences. But AI can also help greatly reduce the impact of human bias. In fact, Limbic Access’s 93% diagnostic accuracy is higher than the average achieved by human clinicians.
The literature on human behavior has defined literally dozens and dozens of distinct and measurable biases: decision fatigue, recency bias, the sunk cost fallacy, the empathy gap, anchoring bias, etc. No one is immune to the human condition.
One of the most significant benefits of safe and properly trained clinical AI is the ability to reduce bias in diagnosis. For example, where a clinician who specializes in treatment for PTSD might naturally overindex on a patient’s mention of the word “trauma,” an AI trained on vast amounts of robust clinical data can more impartially analyze text or language patterns to hone in on underlying issues.
Similarly, over any long discussion—such as an in-depth clinical assessment—humans’ attention naturally ebbs and flows. AI doesn’t experience those cognitive load issues. It analyzes every word and can help clinicians catch things they might otherwise miss.
Race and gender representation
In addition to the behavioral biases listed above, societal biases around race, culture, and gender can also affect not just the quality of care but even who can access it. When people feel judged or victimized, they’re less willing to be vulnerable and discuss things openly, which can lead to misdiagnosis of mental health conditions—and that’s for those who are even willing to seek help in the first place.
As noted above, Limbic Access has been shown to significantly increase the share of individuals from underrepresented groups entering treatment. The fact that AI chatbots are inherently raceless and genderless is highly likely to account for this.
For example, in the Nature Medicine study referenced above, while the absence of human involvement in the Limbic Access chatbot was mentioned as a benefit across all groups, members of gender minority groups were more likely than cis-gendered users to call this out as a specific benefit. They were also more likely to say the assessment experience gave them hope.
Better and Faster Care Pathway Matching
More accurate diagnosis at the assessment stage unlocks faster and better patient-provider matching. And since generative AI is inherently multilingual and always available, it’s better able to serve as a digital front door for diverse populations than even the most well-staffed call centers.
In one peer-reviewed study of 64,862 patients, those assessed via Limbic Access were 45% less likely to need a change to their treatment plans and 18% less likely to drop out of care.
Conclusion
Clinical AI tools like those from Limbic drive better patient outcomes across the board. But the improvements are far greater within historically underserved groups—where there has been much more room for improvement to begin with.
In other words, AI is closing the health equity gap—helping patients feel more welcome in the healthcare system and helping behavioral health services deliver appropriate, effective care to those in need, regardless of background.