Clinical AI for Patients: Separating Signal from Noise
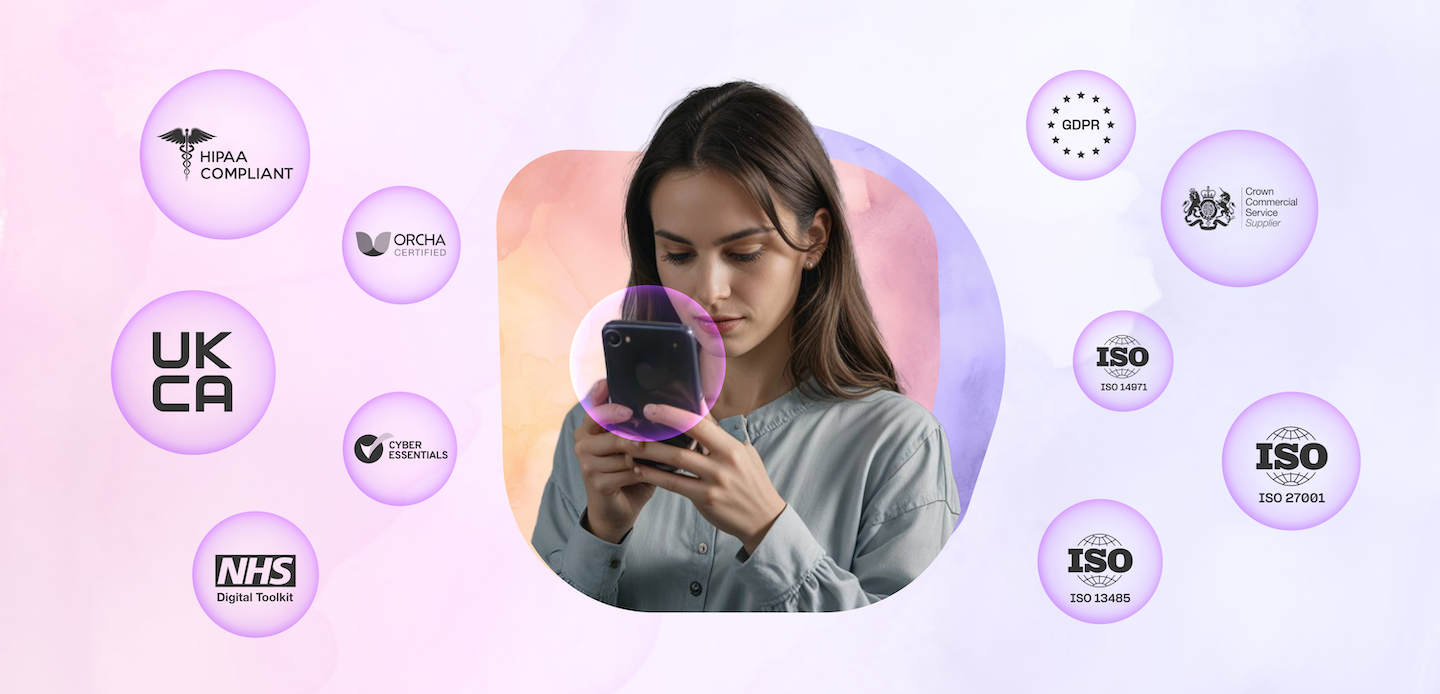
Large Language Models (LLMs) have sparked a gold rush in healthcare, especially in mental health, with seemingly every company claiming their chatbot can revolutionize therapy. It's not hard to see why. Mental healthcare faces a fundamental supply problem: two-thirds of people who need care can't access it. Unlike many medical conditions that can be treated through self-administered medications, psychological therapy inherently requires sustained conversation between patient and clinician. Each hour of therapy represents an irreducible unit of clinical time, creating a natural ceiling on access to care.
LLMs, with their remarkable conversational abilities, are uniquely positioned to help solve this crisis. But as with the dotcom boom before it, this surge of AI 'therapists' has created a turbulent landscape where separating legitimate innovation from dangerous shortcuts isn't straightforward.
The Ethical Argument for LLMs in Patient-Facing Care
To be clear, I am not about to make the tired case that ChatGPT is a replacement for a qualified mental health professional. It’s not. Nor will I downplay the uniquely human qualities of psychological therapy, which fundamentally involves a human experience.
Yet here we are, facing a mental health crisis where two-thirds of people who need care cannot access it. When your house is on fire, you don't reject the fire hose because it's not as elegant as your interior sprinkler system. If LLMs, through their phenomenal conversational capabilities, can scale the impact of clinicians delivering talking therapies, I’d argue that it’s a moral imperative we figure out the path forward.
The real question isn't whether we should use LLMs in patient-facing care; it's how can we do this while ensuring safety and clinical rigor is upheld? This is the ‘hard problem’ of LLMs in healthcare. Addressing it will determine whether AI becomes a genuine force for good or just another overhyped technology.
Avoiding The Hard Problem of Making LLMs Safe
1. “Rule-based” chatbots
A common approach is to limit chatbots to pre-scripted messages written by their creators. First, we should acknowledge that labeling these solutions as “AI” stretches the term considerably. Rule-based systems, by definition, do not demonstrate the adaptive intelligent characteristics of genuine AI. (Skeptics may wonder if the "AI" designation serves more as a marketing tool than an accurate technical description).
By contrast, LLMs produce unique responses tailored to the specific context. Unlike their rule-based predecessors, these systems demonstrate truly intelligent behavior, responding to the nuances of each conversation.
While rule-based chatbots are undeniably safe—primarily because they're largely inert—their fundamental flaw lies in efficacy and usability. Despite the claims of vendors, independent meta-analyses tell a different story: therapeutic effects are small and are not sustained over time. Furthermore, users consistently report frustration with responses that feel empty, generic, nonsensical, repetitive, and constrained.
2. When it’s wellness, not healthcare
In their rush to market, many companies deploying LLMs are sidestepping clinical safety requirements altogether by simply rebranding their tools as "wellness coaches," "AI companions," or other vague labels. By casually dismissing their solutions as non-clinical, they excuse themselves from accountability and responsibility. This sleight of hand is likely to become particularly common among teletherapy providers, who already deliver wider care services, and may wish to quickly add AI features to their offering under the guise of wellness. While legally permissible (for now), it's a dangerous game to play.
The fundamental issue is context: whether labeled a "coach" or not, unvalidated LLM products must not be used within the context of mental health treatment by vulnerable individuals. The tragic suicide of a 14-year-old boy linked to an unvalidated LLM from Character AI highlights the dangers of AI operating without proper clinical oversight and the serious legal and reputational risks for those who fail to prioritize clinical rigor. his isn’t necessarily a question of regulation—the FDA ultimately determines which products fall under medical device oversight. However, any AI tool being used as part of patient care, regardless of its "wellness" branding, must be held accountable to robust peer-reviewed clinical evidence and third party validation of safety.
Addressing the Hard Problem
The path to safe AI in mental healthcare isn't through superficial adaptations of general-purpose LLMs. While these models excel at natural conversation, they fundamentally lack the clinical rigor required for healthcare - their responses are inherently unexplainable, can be unpredictable, and are often inaccurate. These limitations cannot be solved through simple "fine-tuning" or vague notions of "safeguards."
The robust solution is to separate clinical decision-making from conversational prowess. This means developing an independent and specialized clinical reasoning system that works alongside the LLM. Unlike the "black box" of LLMs, this clinical reasoning layer must be trained specifically on healthcare data, explicitly represent established clinical protocols, and provide explainable decisions with quantifiable safety metrics. Think of it as a real-time clinical supervisor, ensuring every LLM interaction adheres to healthcare standards while maintaining the natural conversation quality that makes generative AI so powerful in mental healthcare.
For clarity, this approach is far-removed from simply prompting ChatGPT to "act like a therapist", nor does it boast a wide selection of carefully crafted prompts written by qualified mental health professionals. True progress requires acknowledging that safe mental healthcare AI needs two distinct systems working in concert - one for natural conversation and another for clinical reasoning. Only by cleanly separating these functions can we properly leverage LLMs' conversational strengths while maintaining rigorous clinical standards through dedicated oversight systems.
What Comes Next
In mental healthcare, we face a simple truth: millions need help, and we have a technology that could transform access to care. The temptation to take shortcuts—whether through rule-based systems or unvalidated wellness apps—is understandable but ultimately harmful. True innovation requires tackling the hard problems of safety and clinical rigor head-on. The technology exists. The need is clear.
The question is: will we do the hard work necessary to bridge the gap between LLMs' promise and healthcare's demands?